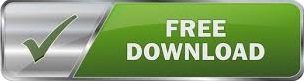
There are also a few more desirable properties for activation functions such as continuous differentiability. In other words, it behaves like a single layer. Usually, it is pointless to generate a neural network for this kind of problems because independent from number of hidden layers, this network will generate a linear combination of inputs which can be done in just one step. However, linear activation functions could be used in very limited set of cases where you do not need hidden layers such as linear regression.
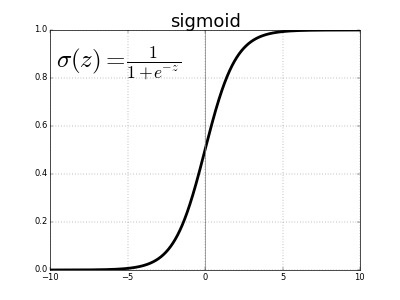
Basically what an activation function does is to generate this non-linearity while mapping input values into a desired range. So we need some functions to generate the non-linearity. In general, real world problems requires non-linear solutions which are not trivial. To understand the logic behind non-linear activation functions first you should understand why activation functions are used. No matter how robust or well hyper tuned your NN is, if you use a linear activation function, you will never be able to tackle non-linear requiring pattern recognition problems In case C happens that means that our classification/prediction problem was most probably a simple linear/logistic regressor based one and never required a neural network in the first place! Therefore, nonlinear functions must be continuous and differentiable between this range.Ī neural network must be able to take any input from -infinity to +infinite, but it should be able to map it to an output that ranges between changes well enough to give us a good fit score

Unfortunately, the small changes occurring in the weights cannot be reflected in the activation values because it can only take either 0 or 1. It is mapped between 0 and 1, where zero means absence of the feature, while one means its presence. An activation function is a decision making function that determines the presence of a particular neural feature. To make the incoming data nonlinear, we use nonlinear mapping called activation function. Input to networks is usually linear transformation (input * weight), but real world and problems are non-linear. However, a non-linear function as shown below would produce the desired results:Īctivation functions cannot be linear because neural networks with a linear activation function are effective only one layer deep, regardless of how complex their architecture is. But as in the second figure below linear function will not produce the desired results:(Middle figure). In short, if the expected output reflects the linear regression as shown below then linear activation functions can be used: (Top Figure). A linear regression aims at finding the optimal weights that result in minimal vertical effect between the explanatory and target variables, when combined with the input.

In fact to understand activation functions better it is important to look at the ordinary least-square or simply the linear regression. > # common activation function, hyperbolic tangentĪ common activation function used in backprop ( hyperbolic tangent) evaluated from -2 to 2:Ī linear activation function can be used, however on very limited occasions. Non-linear means that the output cannot be reproduced from a linear combination of the inputs (which is not the same as output that renders to a straight line-the word for this is affine).Īnother way to think of it: without a non-linear activation function in the network, a NN, no matter how many layers it had, would behave just like a single-layer perceptron, because summing these layers would give you just another linear function (see definition just above).

In turn, this allows you to model a response variable (aka target variable, class label, or score) that varies non-linearly with its explanatory variables The purpose of the activation function is to introduce non-linearity into the network
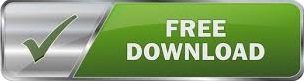